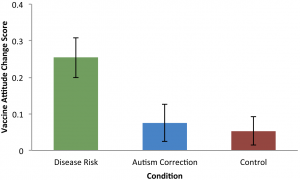
Recently, a number of media outlets enthusiastically reported that “Scare tactics may be the surest way to get parents to vaccinate their children”, suggesting to “Scare the crap out of [anti-vaccine parents]”, and happily claiming that “There’s a surprisingly simple way to convince vaccine skeptics to reconsider”.
Unfortunately, the study that these bold statements are based on, “Countering antivaccination attitudes” by Horne, Powell, Hummel and Holyoak and published in PNAS, suffers from a number of serious flaws. And what is worse, the thinking underlying both the design of the study and the way the media reported on this story misses one of the basics of behavior change - in fact, one of the basic principles of, well, life:
If you want to change something, first learn about it and make sure you understand it.
Before I go into this basic principle, I will point out why the study is flawed. For those pressed in time, this boils down to:
confounded manipulation;
measurement with very low validity;
a small effect size for a dependent variable that very indirectly predict behavior;
the fact that they ignore the past sixty years in fear appeal research;
and most importantly, a missed but crucial step in the process to develop effective behavior change interventions.
[UPDATE: I now go into the validity of their manipulation more in depth in this post.]
The study
The posts I link to above summarize the study, but again for those pressed for time: Horne et al. conducted an experiment with three conditions:
a control condition, receiving a text about bird feeding;
a condition that represented ‘traditional interventions’, where scientific evidence was presented that vaccines do not cause autism, called the Autism Correction condition;
and the new intervention, emphasizing the risks of not getting your children vaccinated, called the Disease Risk condition.
They developed their own measure of attitude towards vaccination, and measured that on the first day of the study. On the second day, those participants that returned were randomized, presented with the intervention of their condition, and completed the attitude measure again. They then conclude that
[t]his study shows that highlighting factual information about the dangers of communicable diseases can positively impact people’s attitudes to vaccination. This method outperformed alternative interventions aimed at undercutting vaccination myths.
How the study is flawed
However, this study does not allow that conclusion - let alone the conclusion that scaring people is the way to get parents to vaccinate their children. The study suffers from a number of serious problems:
The manipulations were not valid
The three conditions vary in a number of ways apart from their main topic (autism vs. risk vs. bird feeding).
First, the word count seriously differs. The Disease Risk condition has 344 words; the Autism Correction condition has 251 words; and the control condition has 193 words. These word counts correlate almost perfectly with the mean change in attitude! I used the brilliant WebPlotDigitizer and entered the image of their anova results on the first page:
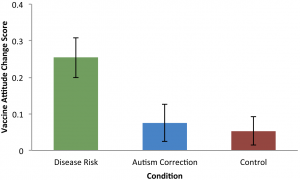
This provided the means for the three conditions: 0.25, 0.07, and 0.05, and used R to correlate these means with the word count for each intervention:
### Enter words and means, compute correlation
<- c(344, 251, 193);
words <- c(0.25, 0.07, 0.05);
means cor(words, means);
[1] 0.9554378
### Compute proportion of explained variance
cor(words, means)^2;
[1] 0.9128614
### Generate plot (note: ggplot2 has to be loaded for this to work!)
::qplot(words, means) +
ggplot2::stat_smooth(method = "lm", se=FALSE) +
ggplot2::ylim(0, .3) +
ggplot2::xlim(0, 500); ggplot2
Warning: `qplot()` was deprecated in ggplot2 3.4.0.
`geom_smooth()` using formula = 'y ~ x'
More than 90% of the variation in the attitude change is predicted by the word count. I wish I found such strong associations in my research . . . But on a more serious note, the difference that was observed could simply reflect the well-known Elaboration Likelihood Model principle that if recipients are not motivated or competent to process a message very thoroughly, their attitude change is determined by peripheral factors such as, indeed, word count . . .
[UPDATE: I now discuss this more in depth in this post.]
Second, the Disease Risk condition is the only one that contained images. Regardless of their content, these images could explain the differences between condition.
Third, the Disease Risk condition contained the following fragment:
Measles, mumps, and rubella (MMR) vaccine can prevent these diseases. Most children who get their MMR shots will not get these diseases. Many more children would get them if we stopped vaccinating.
Those sentences may influence participants’ Response Efficacy, a psychological variable reflecting how effective a behavior is believed to be to alleviate a threat (in this case, how well vaccination works). Enhancing participants’ Response Efficacy could explain the results of this study, even more so because the dependent ‘attitude’ measure actually measures Response Efficacy, among other variables.
The measurement was not valid
The measure of ‘attitude’ that the researchers developed does not measure attitude. It measures a bit of attitude, a bit of intention, a bit of response efficacy, and a bit of something we could call ‘trust in doctors’. All of these bits are operationalised in a way not comparable to previous research. For example, the item “The risk of side effects outweighs any protective benefits of vaccines” combines a number of beliefs as defined by Fishbein & Ajzen (2011). One of the least appropriate questions in the attitude measure is the one that actually measures response efficacy: “Vaccinating healthy children helps protect others by stopping the spread of disease”.
The change cannot be expected to influence behavior
In the caption of Figure 1, Horne et al. (2015) provide the oneway ANOVA results, which allow computation of their effect size, F(2,312) = 5.287, P = 0.006 (see this blog post by Daniel Lakens for the formula):
<- 5.287;
f <- 2;
df1 <- 312;
df2 <- (f - 1) / (f + (df2 + 1) / (df1))
omegasq print(omegasq);
[1] 0.0264978
So, the manipulation (word count, use of images, response efficacy, or something else) explains 2.65% of the variation in the attitude dependent measure. If the dependent measure were behavior, this might even have been acceptable (but see the next paragraph). After all, an intervention that only consists of text and images is quite cheap and easy to distribute very widely. However, the intervention was targeted at Disease Risk. This variable is called Risk Perception, and it forms a part of the psychological variable Attitude. Attitude is generally assumed to influence behavior through Intention. But Behavior is not only predicted by Intention: there are other determinants. Similarly, Intention is not only predicted by Attitude; and finally, Attitude has other components than Risk Perception. Therefore, any change in Risk Perception is watered down considerably before it can hope to influence Behavior (for example, see Webb & Sheeran, 2006). Only explaining 2.65% of Attitude therefore likely translates to no or negligible change in behavior. That is, if Attitude is even changed, because ironically, the behavior change method the authors chose, threatening communication, is notorious for backfiring.
Threatening information usually doesn’t work, and sometimes backfires
Decades of research into behavior change, specifically changing behavior through threatening communication, showed that the theoretical framework used by the authors, subjective utility theory, does not apply to threatening communication. In fact, threatening communication can easily backfire, and even in situations where it does not, it is unlikely to be the most effective behavior change method that is available, as shown by recent meta-analytical evidence (Carey, McDermott, & Sarma, 2013; Peters, Ruiter, & Kok, 2013) and a narrative review compiling sixty years of research into threatening communication (Ruiter, Kessels, Peters, & Kok, 2014). Regarding threatening communication, it is particularly important to measure actual behavior change, as attitude change often does not translate into behavior change. This is explained in depth at this site explaining why threatening communication is generally a bad idea.
In short, the authors do not ‘cleanly’ manipulate Disease Risk; they do not measure attitude change; they find a very small change in a variable that predicts only a tiny part of behavior; and the method they chose is usually ineffective and has a risk of backfiring. But the biggest problem of this study, and the way it was picked up by the media, is much, much worse.
First figure out what to change, then how to change it
The goal of Horne et al. (2015) is very noble, of course, and I completely agree. It is very important to develop effective behavior change interventions to promote vaccination. However, instead of resorting to threatening communication or any other behavior change method and comparing its effectiveness to other methods, it is necessary to first thoroughly understand the problem at hand (Kok et al., 2014). Without a thorough understanding of why some people refuse to vaccinate their children, attempts to attack this problem are shots in the dark. Reasons for refusing childhood vaccination were recently studied in a Dutch sample (Harmsen et al., 2013; the open access publication is here):
Refusal of vaccination was found to reflect multiple factors including family lifestyle; perceptions about the child’s body and immune system; perceived risks of disease, vaccine efficacy, and side effects; perceived advantages of experiencing the disease; prior negative experience with vaccination; and social environment.
If you want to develop an intervention to promote vaccination, why not start with what we know about why people do or don’t get their children vaccinated? Although the situation in the United States will be different, and therefore, different reasons and considerations will predict behavior in the United States, it is unlikely that the situation is so simple that an intervention targeting only risk perception can be effective.
Instead, it is necessary to first identify what should be changed, in the jargon, which ‘beliefs’ and ‘determinants’ predict behavior. I tried to explain how all this works in a relatively accessible open access paper available here (Peters, 2014; also see Kok, 2014, at http://effectivebehaviorchange.com). There are very, very many other tools available for developing effective behavior change interventions, such as the Intervention Mapping protocol (Bartholomew, Parcel, Kok, Gottlieb, & Fernández, 2011).
However, the study by Horne et al. reflect a dangerous tendency: when talking about behavior change, focusing on the change and not first striving to understand the behavior. When asked “How can we change behavior X?”, “How do we get people to quit smoking?”, or “How do we promote exercise among inner-city youth?”, common responses focus on potential strategies to achieve that goal. The response “Well, first let’s make sure we understand the problem” is rarely heard, except from those who have been trained in systematic behavior change. From the perspective of the media, this is understandable. After all, headlines like “Simple intervention can moderate anti-vaccination beliefs, study finds” work better than “Complex evidence- and theory-based multi-component intervention achieves small increase in vaccination behavior”. From researchers or intervention developers, this is a bit harder to understand. After all, they have access to literature where protocols for intervention development are described (like the Intervention Mapping protocol I just mentioned).
Perhaps a part of the problem is that behavior change science is a part of psychology - and everybody’s a psychologist. When two partners are discussing the behavior of their children, they use their common sense to figure out how they should intervene: and similarly, many people use their common sense to think about how to intervene with the behavior of other people. They’re people, after all - they’re just like us. And if you didn’t study psychology, you don’t know that people are often not rational (but almost always think they are), that people often apply very economical but easily misguided heuristics, and that many processes exist to maintain a positive self-image rather than behave in the most optimal way. There does seem to exist a tendency to discuss other people’s behavior based on the assumption that the products of introspection are informative as to others’ mental landscapes.
This is consistent with the outcomes of a recent qualitative study into why fear appeals remained such a popular weapon of choice for intervention developers, politicians, policymakers, and even some scientists (Peters, Ruiter & Kok, 2014). For example, one scientist who was interviewed said:
I would, if I would read, like, “It gives you lung cancer”, then I would stop to think, like, “Well, I don’t think this is such a nice idea.”
And a politician said:
[…] they showed a brain scan with pictures. So one picture of a child who had not consumed alcohol, and another scan of a child who had consumed alcohol. And showed what it does to your brains. Well, they showed that at the meeting, and then people could ask questions. And then I thought, I think that works, because people then start thinking.
It appears there is a strong (and understandable) tendency to assume that introspection is a useful tool for understanding others. However, psychologists of all people should know better. In addition to the decades of research on behavior change methods (which have shown, for example, that threatening people is usually a bad idea), we also have decades of research on understanding behavior, and it’s time we start combining all of this in our efforts to promote health and in this case, vaccination.
[UPDATE: I now go into the validity of their manipulation more in depth in this post.]
Notes
You can take a look at the measurements and interventions yourself if you want - Horne et al. made their measurements and manipulations publicly available (which is very commendable! I wish they’d also made their data available).
References
Bartholomew, L. K., Parcel, G. S., Kok, G., Gottlieb, N. H., & Fernández, M. E. (2011). Planning health promotion programs: an Intervention Mapping approach (3rd ed.). San Francisco, CA: Jossey-Bass.
Carey, R. N., McDermott, D. T., & Sarma, K. M. (2013). The impact of threat appeals on fear arousal and driver behavior: a meta-analysis of experimental research 1990-2011. PloS One, 8(5), e62821. doi:10.1371/journal.pone.0062821
Fishbein, M., & Ajzen, I. (2010). Predicting and changing behaviour: The Reasoned Action Approach. New York, NY: Taylor & Francis.
Harmsen, I. A., Mollema, L., Ruiter, R. A. C., Paulussen, T. G. W., de Melker, H. E., & Kok, G. (2013). Why parents refuse childhood vaccination: a qualitative study using online focus groups. BMC Public Health, 13(1), 1183.
Horne, Z., Powell, D., Hummel, J. E., & Holyoak, K. J. (2015). Countering antivaccination attitudes. Proceedings of the National Academy of Sciences, 201504019. doi:10.1073/pnas.1504019112
Kok, G. (2014). A practical guide to effective behavior change: How to apply theory- and evidence-based behavior change methods in an intervention. European Health Psychologist, 16(5).
Kok, G., Bartholomew, L. K., Parcel, G. S., Gottlieb, N. H., Fernandez, M. E., & Fernández, M. E. (2014). Alternatives to fear appeals: Theory- and evidence-based health promotion, an Intervention Mapping approach. International Journal of Psychology, 49(2), 98–107. doi:10.1002/ijop.12001
Kok, G., Gottlieb, N., Peters, G.-J. Y., Mullen, P. D., Parcel, G. S., Ruiter, R. A. C., … Bartholomew, L. K. (2015). A taxonomy of behavior change methods: an Intervention Mapping approach. Health Psychology Review.
Peters, G.-J. Y. (2014). A practical guide to effective behavior change: how to identify what to change in the first place. European Health Psychologist, 16(4), 142–155.
Peters, G.-J. Y., Ruiter, R. A. C., & Kok, G. (2013). Threatening communication: a critical re-analysis and a revised meta-analytic test of fear appeal theory. Health Psychology Review, 7(Suppl 1), S8–S31. doi:10.1080/17437199.2012.703527
Peters, G.-J. Y., Ruiter, R. A. C., & Kok, G. (2014). Threatening communication: A qualitative study of fear appeal effectiveness beliefs among intervention developers, policymakers, politicians, scientists and advertising professionals. International Journal of Psychology, 49(2), 71–79. doi:10.1002/ijop.12000
Ruiter, R. A. C., Kessels, L. T. E., Peters, G.-J. Y., & Kok, G. (2014). Sixty years of fear appeal research: Current state of the evidence. International Journal of Psychology, 49(2), 63–70.
Webb, T. L., & Sheeran, P. (2006). Does changing behavioral intentions engender behavior change? A meta-analysis of the experimental evidence. Psychological Bulletin, 132(2), 249 –268. doi:10.1037/0033-2909.132.2.249